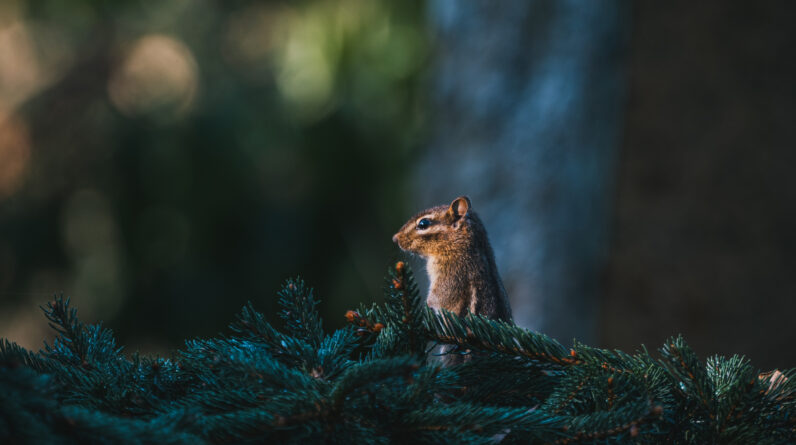
So, you want to know how to conduct A/B testing for your ad campaigns? Well, you’re in the right place! In this article, we’ll explore the ins and outs of A/B testing and provide you with a comprehensive guide on how to run effective experiments to optimize your advertising efforts. From understanding the importance of A/B testing to analyzing the results, we’ll cover all the essential steps you need to take to ensure your ad campaigns reach their full potential. Get ready to unlock valuable insights and take your advertising game to the next level! A/B testing is a powerful tool used by marketers to optimize their ad campaigns and improve conversion rates. It involves comparing two different versions of an element, such as an ad, to determine which one performs better. In this article, we will explore what A/B testing is, its benefits, and when to use it. We will also provide a step-by-step guide on how to conduct A/B testing for ad campaigns, including setting clear objectives, creating variations, determining sample size, randomizing test groups, implementing tracking mechanisms, running the test, monitoring and analyzing results, optimizing based on insights, and considering best practices.
What is A/B Testing?
Defining A/B testing
A/B testing, also known as split testing, is a method used to compare two different versions of an element to determine which one produces better results. In the context of ad campaigns, A/B testing involves comparing different variations of ads to understand which version drives higher engagement, conversions, or other desired outcomes. By conducting A/B testing, marketers can make data-driven decisions to optimize their campaigns and achieve better results.
Benefits of A/B testing
A/B testing offers numerous benefits for ad campaigns. It allows marketers to understand which elements of their ads are resonating with their target audience, identify areas of improvement, and optimize their campaigns accordingly. By testing different variations, marketers can eliminate guesswork and rely on data to make informed decisions. This not only leads to better campaign performance but also helps in allocating resources effectively and maximizing return on investment (ROI).
When to use A/B testing
A/B testing can be applied at various stages of an ad campaign and can be beneficial in multiple scenarios. It can be used when launching a new ad campaign to determine which creative, messaging, or design resonates better with the target audience. A/B testing can also be useful when making changes to an existing campaign, such as testing different headlines, images, or calls to action. Furthermore, A/B testing can be employed to optimize landing pages or email campaigns, allowing marketers to refine their strategies based on real-time data.
Setting Clear Objectives
Identifying campaign goals
Before conducting an A/B test, it is crucial to clearly define the goals of the ad campaign. What specific outcomes or actions do you want to achieve? Is it increased click-through rates, higher conversion rates, or improved customer engagement? Having well-defined goals will help guide your A/B testing strategy and enable you to measure the success of different variations accurately.
Establishing key metrics
Once you have identified your campaign goals, it is essential to establish key metrics that align with those objectives. These metrics will serve as benchmarks to compare the performance of different variations. For example, if your goal is to increase click-through rates, the key metric could be the number of clicks generated by each ad version. By selecting relevant and measurable metrics, you can evaluate the success of your A/B test and make data-driven decisions to optimize your ad campaign.
Creating Variations
Understanding the control group
When conducting an A/B test, it is important to have a control group against which you can compare the performance of your variations. The control group represents the current version or existing state of your ad campaign. It serves as a baseline against which you can measure the effectiveness of the alternative versions. By understanding the control group’s performance, you can identify areas for improvement and optimize your campaign accordingly.
Developing alternative ad versions
To conduct an A/B test, you need to create alternative versions of your ad. These variations should differ only in the element you want to test, such as the headline, image, or call to action. Keeping other elements constant will ensure that any differences in performance can be attributed to the specific variation being tested. Creating multiple variations allows you to compare the impact of different elements and identify the most effective combination for your ad campaign.
Determining Sample Size
Calculating statistical significance
To ensure that your A/B test results are reliable and meaningful, it is important to determine the appropriate sample size. Statistical significance refers to the probability that the observed results are not due to chance. By calculating the statistical significance of your test, you can determine whether the differences in performance between variations are statistically significant or simply random occurrences. This helps in making confident decisions based on the data collected during the A/B test.
Considering confidence level and power
In addition to calculating statistical significance, it is important to consider the confidence level and power of your A/B test. The confidence level represents the degree of certainty you have in the results of your test. A higher confidence level, such as 95% or 99%, indicates a greater level of confidence in the observed differences between variations. Power, on the other hand, refers to the probability of correctly rejecting the null hypothesis when it is false. By considering both confidence level and power, you can ensure that your A/B test is robust and provides reliable insights.
Randomizing Test Groups
Ensuring unbiased allocation
To obtain accurate and unbiased results from your A/B test, it is crucial to randomly allocate participants or traffic to different variations. Randomization helps in reducing the impact of confounding factors and ensures that the groups being tested are comparable. By randomly assigning participants or traffic, you can minimize the influence of external variables that could skew the test results. Randomization is a fundamental principle of experimental design and is essential for obtaining valid insights from your A/B test.
Using randomization techniques
There are various randomization techniques that can be used to allocate participants or traffic to different variations. Simple randomization involves randomly assigning participants without any specific criteria or stratification. Stratified randomization, on the other hand, involves dividing participants into homogeneous subgroups and then randomly assigning them to variations. This technique ensures that each subgroup is represented in all variations, allowing for more accurate comparisons. Randomization techniques play a crucial role in reducing bias and ensuring the validity of your A/B test results.
Implementing Tracking Mechanisms
Utilizing tracking pixels
To track and measure the performance of your ad variations, it is essential to implement tracking mechanisms such as tracking pixels. Tracking pixels are small, transparent images or code snippets that are embedded in the ad or landing page. They allow you to collect data on user interactions, such as clicks, conversions, or page views. By utilizing tracking pixels, you can accurately measure the performance of each variation and gather valuable insights for optimization.
Implementing UTM parameters
In addition to tracking pixels, implementing UTM (Urchin Tracking Module) parameters can provide valuable insights into the performance of your ad variations. UTM parameters are tags or strings that are added to the URL of your ad. They allow you to track the source, medium, campaign, and other relevant information about the ad. By including UTM parameters in your ad URLs, you can track and analyze the effectiveness of different variations across different channels and campaigns.
Running the A/B Test
Defining test duration
When running an A/B test, it is important to define the duration of the test carefully. The test duration should be long enough to collect a sufficient amount of data for analysis but not too long that it becomes impractical or impacts campaign performance. The ideal test duration will depend on factors such as the size of your target audience, expected conversion rates, and the level of statistical significance you want to achieve. By defining the test duration appropriately, you can ensure that your A/B test provides meaningful insights without disrupting your overall campaign strategy.
Splitting traffic evenly
To conduct a fair A/B test, it is crucial to split the traffic evenly between different variations. This means that an equal number of participants or visitors should be assigned to each ad version. Splitting traffic evenly helps eliminate biases and ensures that each variation receives an equal opportunity to perform. By distributing traffic evenly, you can obtain accurate and reliable results, enabling you to make informed decisions regarding the optimization of your ad campaign.
Monitoring and Analyzing Results
Tracking key metrics
During the A/B test, it is essential to track and monitor the key metrics established in the earlier stage. This allows you to measure the performance of different variations and identify patterns or trends. By monitoring key metrics, you can gain insights into the effectiveness of each variation and determine which one aligns best with your campaign goals. Tracking metrics throughout the test duration enables you to make data-driven decisions and optimize your ad campaign based on real-time feedback.
Comparing performance
Once the A/B test is complete, it is time to compare the performance of different variations. Analyzing the collected data and comparing key metrics will help you identify which ad version performed better in terms of the desired outcomes. Look for statistically significant differences and consider other factors such as the confidence level and power of your test. By comparing performance, you can select the winning variation and make informed decisions about the optimization of your ad campaign.
Evaluating statistical significance
When comparing the performance of different variations, it is important to evaluate the statistical significance of the observed differences. Statistical significance helps determine whether the observed differences are likely to be due to the variations being tested or simply due to chance. By taking into account the sample size, confidence level, and power of your test, you can evaluate the statistical significance of your results. This ensures that the differences observed are meaningful and can be relied upon for making data-driven decisions.
Optimizing Based on Insights
Identifying winning variations
Based on the results and statistical significance of your A/B test, you can identify the winning variations. These are the ad versions that performed significantly better in terms of your campaign goals and key metrics. By identifying the winning variations, you can understand which elements of your ads resonate best with your target audience. This can help guide future ad campaigns and enable you to optimize your current campaign based on data-driven insights.
Implementing changes
Once you have identified the winning variations, it is time to implement the changes in your ad campaign. This may involve updating the creative, messaging, design, or other elements based on the insights gained from the A/B test. By implementing changes, you can improve the overall performance of your ad campaign and achieve better results. It is important to track the impact of these changes and continue monitoring key metrics to ensure that the optimizations are effective.
Continuing iterations
A/B testing is an ongoing process that requires continuous iterations and improvements. Even after implementing changes based on the insights gained from a single A/B test, it is important to continue testing and optimizing. By constantly experimenting with different variations and monitoring the results, you can refine your ad campaigns and achieve continuous improvements in performance. A/B testing is a valuable tool for iterative optimization and can significantly enhance the success of your ad campaigns.
Considerations and Best Practices
Test one variable at a time
To accurately measure the impact of different elements, it is important to test one variable at a time. By isolating variables and testing them individually, you can determine the specific influence of each element on the performance of your ad campaign. Testing multiple variables simultaneously can lead to confounding effects and make it difficult to attribute changes in performance to a specific element. By testing one variable at a time, you can gain precise insights and optimize your ad campaign more effectively.
Avoid inconsistent testing periods
When conducting A/B tests, it is crucial to avoid inconsistent testing periods. Inconsistent testing periods can introduce biases and make it challenging to compare the performance of different variations accurately. It is important to run the A/B test for a consistent duration across all variations to ensure fair comparisons. By maintaining consistency in testing periods, you can obtain reliable and meaningful insights that can be used to optimize your ad campaigns.
Leverage historical data
While conducting A/B tests, it is beneficial to leverage historical data to inform your decisions. Historical data provides insights into the performance of previous ad campaigns, allowing you to identify trends, patterns, and successful strategies. By leveraging historical data, you can make more informed decisions regarding the elements to test and the variations to create. Historical data serves as a valuable resource for optimizing your ad campaigns and achieving better results.
In conclusion, A/B testing is a powerful technique that enables marketers to optimize their ad campaigns based on real-time data and insights. By setting clear objectives, creating variations, determining sample size, randomizing test groups, implementing tracking mechanisms, running the test, and analyzing the results, marketers can make data-driven decisions and continuously improve the performance of their ad campaigns. By following best practices such as testing one variable at a time, avoiding inconsistent testing periods, and leveraging historical data, marketers can maximize the effectiveness of their A/B tests and achieve better results in their ad campaigns.